Ever heard of the Christmas Tsunami? Or the Boxing Day Tsunami?
Well, most people associate these days with good memories, cheerful carols, and spending time with loved ones but not so for the more than 200,000 people killed by a 9.1 magnitude tsunami off Sumatra Island, Indonesia. The tsunami ruptured a 900-mile stretch fault at the convergence point of the Australian and Indian tectonic plates. In geographical terms, the tsunami occurred after a heavy ocean plate slipped under a lighter continental plate resulting in a megathrust quake. After eight hours and more than 5,000 miles from its Asian epicentre, the tsunami earthquake claimed its last casualties on the South African Coast. While tsunami scientists continue gaining massive insight and knowledge to make objective predictions, most data become available long after the damage has occurred.
The Value of Data
Making tsunami forecasts hinges on different factors. Normally, it requires real-time testing of water level networks, bathymetry, topography, and preset scenarios from the seismic paradigm to assess the possible movement of the tsunami across the ocean and its impact on certain coastal areas. Derived elements include wave height and expected arrival time, the longevity of the tsunami plus location and severity of coastal flooding. To predict a tsunami, any methodology must avail data in a trusted and actionable manner to help scientists save lives and mitigate possible damages.
Researching Tsunamis
People often ask how scientists conduct research on tsunamis. In most instances, the intent remains to understand how to acquire a clear, standard, and acceptable method to predict tsunamis. Historically, this is a tall order despite technological advancements.
However, here are a few technological methods making headways in forecasting tsunamis.
Deep-Ocean Tsunami Detection Buoy
Aims to confirm the existence of tsunami earthquakes caused by undersea earthquakes to ensure real-time reporting before these quakes reach the surface. Typically, a tsunami detection buoy consists of two elements; the surface buoy and the pressure sensor anchored on the sea floor. The latter traces changes in water pressure by measuring any variation in the water height column. Through acoustic telemetry, the water height column is recorded by the surface buoy and communicated to scientists using satellite imaging.
The system applies operates on the standard and event mode. In most instances, the standard mode is commonly used to routinely collect sea level information and relay the same via satellite at low interval frequencies, usually every 15 minutes. This approach helps save battery and allows longer deployment periods. Faster moving seismic wave movement detected by pressure sensors through the sea floor triggers the ‘event’ mode, which then begins at one-minute intervals to objectively ascertain whether a tsunami will occur. If the rapid movement turns out to be a false alarm, the intervals alternate after 4 hours.
Cab Supercomputers and Artificial Intelligence predict tsunamis?
Following the loss of 20,000 people in Japan due to the devastating impact of the Christmas Tsunami, the government sought to rectify the unreliability of the Early Warning System in place (EWS). Instead of reporting the wave height at 50m, the EWS underreported the wave height as 3m and thus downplaying the severity of the tsunami.
Japan’s Fujitsu Laboratories built an AI model aimed at estimating coastal flooding following tsunami earthquakes in real-time. The scientists used Fugaku, the world’s fastest supercomputer, to develop the model. A team of tsunami researchers collected training data for 20,000 tsunami scenarios based on high-resolution resolutions generated by the supercomputer and then built an AI algorithm from this database.
The algorithm showed how in the event of an earthquake, the resultant tsunami waveform data observed the offshore flooding impact before the wave hits land. With the data, scientists anticipate the level of flooding and damage to infrastructure and human activities from the localised waves. Evacuation plans and other disaster mitigation plans can be duly undertaken.
Additionally, the model runs seamlessly on standard PCs which makes the creation of real-time data possible.
Machine Learning Algorithms for Tsunami Forecasting
A research paper accessible on Science Reports reported how scientists at Cardiff University are spearheading the next frontier of tsunami predictions based on machine learning. The researchers say their analysis of ocean soundwaves triggered by underwater quakes helped them design the algorithm.
By applying acoustic signals, the researchers hope to understand the type of earthquake and retrieve its main properties. In its development phase, the research collected deep sound recordings of 201 earthquakes that took place in the Pacific and the Indian Ocean. Tsunamis mostly occur after vertical quakes since the tectonic plates on the earth’s surface move up and down rather than horizontally. The vertical motion displaces large amounts of water, creating long waves with the capacity to cause widespread onshore damage.
The vertical motions compress the water layer which in turn sends sound signals with information on the geometry and dynamics of the fault. The research team trained machine learning to identify the occurrence of a vertical earthquake, which mostly precedes the eventuality of a tsunami.
Tectonic movements are complicated with vertical and horizontal traits. An earthquake’s ability to cause a tsunami differs. With an AI model to analyse the underwater quakes, the moment magnitude is recorded and the possibility of a tsunami (in)validated.
Time Reverse Imaging Method – Retracing Tsunamis to forecast tsunamis in the future
It’s a method that recreates images from ocean sensors to visualise how a tsunami appeared at ‘birth’. The method departs from reliance on region-specific patterns for predicting tsunamis. Relying on past trends can be devastating if unknown patterns arise. The algorithm gathered data from the Tohoku-Oki tsunami of 2011 to form its foundation. By mathematically going back on how initial sea displacement took place compared to the resultant imaging, the algorithm refined its accuracy. Researchers aim to replicate the algorithm on other tsunamis to further improve its accuracy before deployment.
Challenges in Tsunami Predictions
A recent model using the buoy model went further by incorporating a Global Positioning System (GPS) and digital compasses to acquire roll, pitch, and heading information. This model captures side-to-side motion to assess tsunami-causing traits. Unfortunately, the model only works in the deeper waters, 100 metres and above, where there is minimal noise.
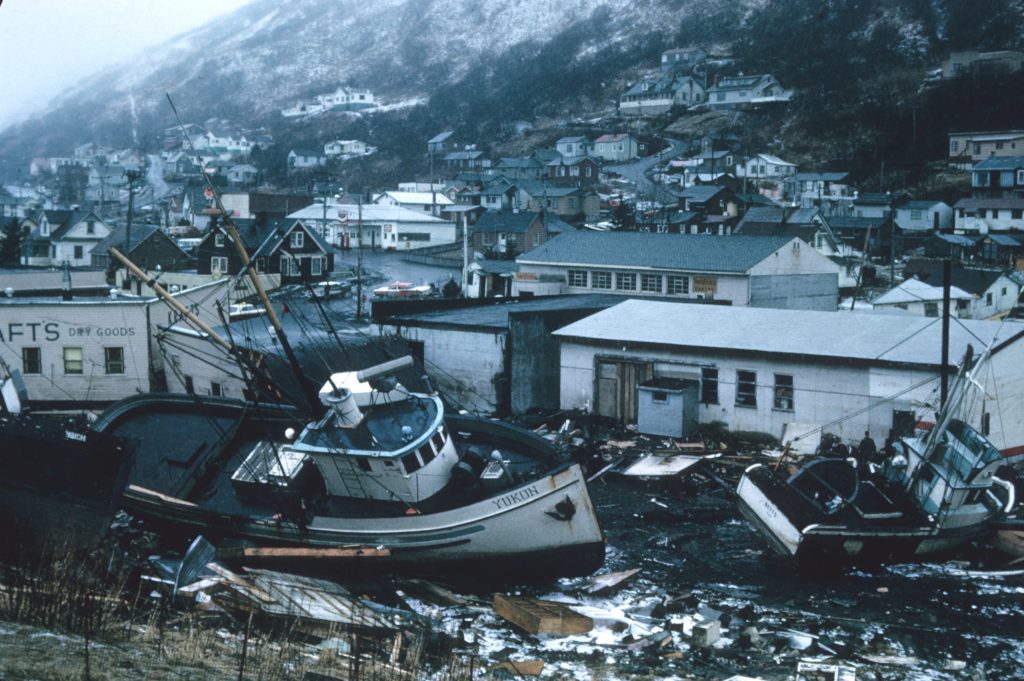
Sending false alarms is one of the key challenges facing most tsunami prediction models. For instance, since Hawaii’s Pacific Tsunami Warning Centre establishment in 1948, about 75% that led to massive evacuation costs and other mitigation efforts turned out to be false. Overcoming this problem is elusive but governments planted pressure sensors on sea floors connected to a cable stretching from the coastline. Some states such as Oregon have laws that prohibit the building of critical infrastructure in areas marked as tsunami inundation zones.
A Cumulative Tsunami Software is Needed
Combining the existing tsunami software is a priority moving into the future. Tsunami technology, summarized as Tweb (evaluates tsunami source scenarios), TsuCAT (supercomputer simulations), and Short-term Inundation Forecasting for Tsunamis (SIFT) which indicates real-time tsunami data on specific coastal regions must be consolidated.
Our collective hope is that Christmas brings cheers and good memories to all; including people living in tsunami-prone areas. It all starts with developing a valid, actionable, and infallible tsunami prediction tool. The good news is that the progress is encouraging.