Eastern Afghanistan cries for our collective help. With more than 1000 people confirmed dead and more than 1500 injured, the region bleeds. CNN reports a 5.9 magnitude hit in the region with the impact felt in neighbouring countries Pakistan and India. Such is the devastating impact of earthquakes yet a prediction model remains a long way mark.
Earthquake forecasting
Indisputably, modern scientists understand earthquakes better than they did decades ago. However, the United States Geological Survey (USGS) categorically states “Neither the USGS nor any other scientists have ever predicted a major earthquake. We do not know-how, and we do not expect to know how any time in the foreseeable future” and this further complicates prospects of preventing major earthquake disasters.
Currently, earthquake forecasting calculates the probability of a significant earthquake taking place in a certain region in a number of years.
Definitive Earthquake Predictions
Some people claim they can predict earthquakes. That’s debatable. An objective earthquake prediction must meet three criteria: provide date and time, the location, and magnitude. Herein lies the key reasons why earthquake scientists dispute such claims.
- Inability to define the three criteria required to make an accurate prediction.
- Such predictions aren’t backed by scientific evidence yet earthquakes are a consequence of scientific processes but not subjective aspects such as slug, rain, or body aches.
- Generalized earthquake predictions conveniently fit into a specific range. For instance, when one talks of an earthquake happening in the Dover Straits.
Machine Learning and Earthquakes
Do you know more than 200,000 earthquakes are recorded globally each year? Interestingly, earthquake scientists believe more than 2 million earthquakes take place. It highlights the huge gap in coming to a conclusive and standard procedure for predicting, monitoring, and mitigating earthquakes. Fortunately, over the past decade, machine learning continues to be adopted in seismological studies.
Under the earthquake machine learning model, the foundation lies in the detection of workflows. These include phase association, location, detection, arrival time measurement, and characterization. With the machine learning approaches, all these elements have witnessed rapid progression. The availability of large labelled data sets, often easily accessible, and methodically constructed by special analysts is an opportune factor.
The data sets precede the launching of a complex supervised earthquake machine. Seismic research is conducted after earthquakes take place, such as the one in Afghanistan, and then machine learning is deployed in an operational mode for real-time monitoring. The goal is to produce next-generation earthquake catalogues with extensive information on one or more earthquake factors complete with high-resolution pictures of seismically active faults.
These next-generation earthquake catalogues will not be single and static objects commonly used by seismologists. For instance, after the Ridgecrest, California earthquake in 2019, the earthquake scientists built four next-generation catalogues, each carrying different enhanced detection techniques. The idea remains to keep updating earthquake catalogues and dramatically improved with time.
Deep learning models, mainly second generation, are designed to mimic manual earthquake characteristics manually developed by analysts. Studies show they offer more value and accuracy, unlike the past models that applied neural network architectures borrowed from other fields. What’s apparent is the expected refining of machine learning by earthquake scientists.
Omori’s Law
Earthquake physics is intricate. A Japanese seismologist after whom this law is named, formulated scientific observations on the decay aftershocks back in the 1890s. According to Omori’s Law, the rate of aftershocks decays with time after the mainshock and these decays happen to be inversely proportional to the time after the main shock. The law is denoted as:
N(t) ∝ (c+t)-p where
N represents the number of aftershocks as a function of t (time) proceeding the mainshocks while c and p are constant. Usually, the constants are >1.
Under this law, the Epidemic Type Aftershock Sequence (ETAS) model was founded. It exists on the premise earthquake is a self-exciting process due to their frequency of occurrence and Gutenberg–Richter statistics for their magnitude. The utility of these laws constantly changes and direct attempts to solve the seemingly stubborn challenge of earthquake forecasting. The ETAS model is applicable in predicting large earthquakes in a sequencing paradigm based on time dependence.
ShakeAlert and Artificial Intelligence Attempts
On the US West Coast, a more traditional computing system is already in use. The model is known as the ShakeAlert. It mainly functions by detecting the first earthquake-triggering waves – popularly known as P waves – and then calculating how the more severe and destructive but slow-moving S waves are likely to arrive.
A new system in incubation is the DeepAlert which aims to build algorithms aimed at providing a few seconds of warning of any expected shaking before an earthquake strikes. The DeepAlert relies on deep neural networks, a form of AI learning, to highlight previous patterns from earthquakes to make predictions on the travelling path of new earthquakes. The goal is to create a standard measure of forecasting and monitoring earthquakes across earthquake-prone areas.
Sobering Truths
Seismologists agree the goal of earthquakes forecasting is not to detect slow slips but rather to predict abrupt, severe, and destructive earthquakes. However, there have been mixed results. The machine learning approach lacks adequate data since catastrophic earthquakes are rare. The problem is the collection of data to modify the algorithms to forecast such quakes. Where do they find such data if the quakes are infrequent?
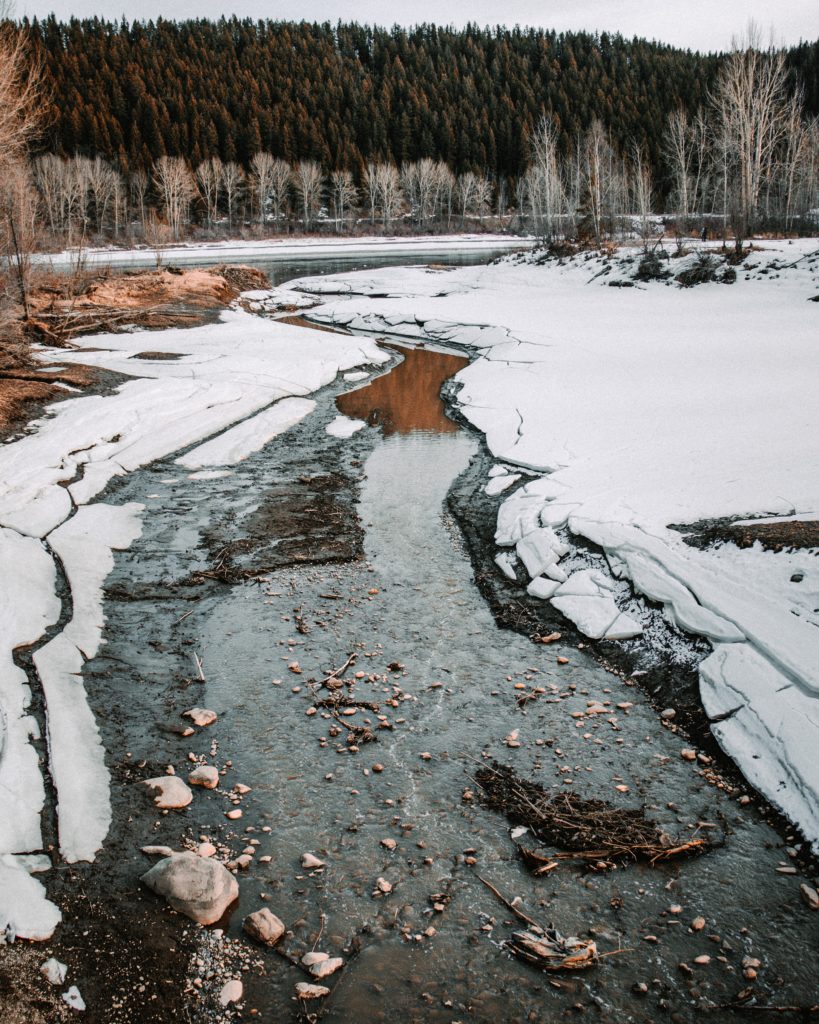
Encouragingly, some studies indicate a statistical correlation between small earthquakes and bigger ones. Therefore, a computer model with algorithms modified to detect these smaller earthquakes could objectively predict larger earthquakes. Machine learning offers hopes of finding the right simulations to mimic fast earthquakes deployable to the real world.
Truth is, seismologists contend with randomness as an element of most earthquakes. Predicting the scientific processes that push a fault to the brink of a rupture is inadequate to correctly evaluate and pinpoint the exact moment it happens. Timing remains a mirage in most earthquake predictions. The best-case scenario is the provision of earthquake predictions in ranges, say within a specific week, month or year. Mass evacuations and disaster mitigation would be difficult to plan under such circumstances.
Earthquake Machine MythBusters
The Tesla earthquake machine is folklore among seismologists. In 1896, Nikola Tesla built an alternating current system with the capacity to transmit electricity across long distances. When testing the machine in his lab at 48 E. Houston St., New York, the resultant vibrations were so huge that concerned neighbours contacted police thinking it was an earthquake. Bemused at their reaction, Tesla simply pocketed his ‘earthquake machine’ and walked away.
Fun Fact: Follow the Male Toads
Despite having all machinery and intelligence, scientists cannot match the accuracy of male toads (Bufo bufo) in predicting earthquakes. A 2010 study carried out by the Journal of Zoology found that male toads abandoned their breeding sites before a major earthquake struck L’Aquila, Italy, in 2009, about 46 miles (74 kilometres) away. Researchers remain puzzled at how male toads manage to do it.
Forecasting earthquakes is an arduous task unless you’re a male toad but the scientists work round the clock hoping to crack it. Hope springs eternal.