Technology is indisputably the next level of driving policy and business decisions. For ages, the normalisation of weather, financial, and market forecasts suffocated the applicability and acceptability of technology to influence the future. Despite its relative infancy, technology forecasting must start gaining more prominence than currently provided.
History of Technology Forecasting
A forecast comes from a deeper analysis of data extraction techniques and interpretation of data. In most instances, there exists a variation in data collection and generating objective conclusions. After World War II, the US government developed frameworks to identify areas with the greatest promise to military necessity in the future. Then in 1945, a report entitled Toward New Horizons ushered the era of using technology predictions.
Characteristics of Credible Data Trends
When it comes to the integration of technology in predictions, two thresholds must be attained: expertise and statistical validity.
The use of technology to predict trends heavily relies on statistical paradigms and hence the need to meet these traits.
- Currency: One cannot provide a prediction based on stale data. Data timelines must coincide with the scope and relevancy of the prevailing situations. While historical data is critical, its integration into modern fields such as information technology is minimal.
- Extraction framework: If one uses shaky and dubious methods to generate data, it undermines the whole process and any conclusions.
- Bias: In the technology field, different businesses and policymakers have different interests to protect hence the need to ensure objectivity is maintained.
- Wholesomeness: Can one understand the thought processes, methodologies involved, and overall conclusions from the process?
Pro tip
Technology trends, and all other forms of predictions, should not be judged on accuracy since it’s possible to generate poor forecasts from credible data collection methods.
Technology Forecasting Methods
Normally, technology forecasting methods are classified into four main methods.
Extrapolation and Trend Analysis
Relies on evaluating past insights to decipher future developments. The method assumes the future represents a rational extension of the past. Through identification and extrapolation of relevant trends, predictions can be made. This approach proves effective in some aspects but causative factors to the trends under review must be considered. Any significant dynamism in the causative factors affects the viability of the trends.
There are some methods that rely on historical data.
- Trend Extrapolation
The technology uses data sets to identify relevant data systems extendable over time to determine capability. It encourages tracking changes in interests to gather insights. Moore’s law argues the historical rate of computer improvement in processing capability predicts future performance.
- Gompertz and Fisher-Pry Substitution Analysis
Hinged on the observation technologies use a certain trend as they are deployed, developed, and reach market maturity and saturation. Such a trend is denoted as the S-curve (Reference). Under the technology trends framework, the above methods determine when products are almost reaching maturity and likely to be overhauled by new technology.
- Morphological Analysis (TRIZ)
Aims to comprehend the evolution of technology over time and its impact on future development. Using the technological evolution laws, the method describes the dynamism of technology depending on innovation and various factors. It projects the future traits of technology through a structured process guided by the Laws of Technological Evolution resulting in new products, software, and technologies.
- Analogies
Identifies past situations and technologies based on recent interests and applies historical data to highlight future projections. Research indicates “The accuracy of this forecasting technique can be improved by using a structured approach to identify the best analogies to use, wherein several possible analogies are identified and rated with respect to their relevance to the topic of interest.”
Judgmental and Intuitive Models
Remember when Bill Gates, the Microsoft CEO, talked about the danger of a looming pandemic? Suffice it to say, the COVID-19 pandemic struck and the world was caught off-guard. That’s the power of opinions to relay forecasts. The judgmental paradigm depends on the ingenuity of an expert to apply their intuition to predict. Former Apple CEO, Steve Jobs believed in the viability of smartphones and built the most valuable smartphone company.
Group forecasts can be majorly influenced by the outspokenness, charm, or soft power exercised by one person. Additionally, personalities are easy to manipulate, and this led to the development of more structured group predictions, such as the Delphi method.
The Delphi Method
Works on eliciting forecasts from a group of experts through consensus-building. Three key attributes: controlled responses, statistical group feedback, and anonymity guide the process to minimise the effect of any interactions. Questionnaires are sent to the team of experts and a summary response of forecasts is produced. A follow-up questionnaire with the summary asks for the need to alter the initial response based on insights from the summary. The result is repeated until a certain consensus criterion is achieved.
Models
Technology forms a set of equations to describe a physical phenomenon. Also referred to as the causal model since available information is gathered to predict the future. Computers and other tech devices provide room for complex models but the inability to account for socioeconomic changes undermines the process. Interestingly, the forecasts matter less compared to trends revealed and responses to inputs and assumptions.
It’s used in forecasting disruptive technology and includes the following methods.
Influence Diagrams
A compact, graphical and arithmetical representation of a decision situation. The cause-and-effect relationship is related to critical risks/uncertainties leading to a decision as shown by an interconnection graph. Basically, these are refined versions of decision trees.
Theory of Increasing Returns
The law of diminishing returns argues that the more a product becomes common, the marginal opportunity cost (foregoing one more unit for the next best alternative) significantly increases. One need not look further but at the evolution of the DVD players.
Businesses no longer follow conventional business laws. The law of increasing returns states that with a proper network, the already successful continue to be more successful. When companies receive a positive response from the market for a newly-launched product, it’s awarded a ‘lock-in’ on Google and other influential spaces. For a new product to replace an old product from the market, it has to offer significant utility but this isn’t common in the goods market. Disruptive technology trends don’t equate to dislodging existing products since an array of factors come into play.
Artificial Neural Network and Chaos Theory
According to Wang 1999, “Technology evolution can be modeled as a nonlinear process exhibiting bifurcation, transient chaos, and ordered state.” It means that the future performance of a product follows a repetitive rather than a linear paradigm. Under the same breath, the theory portends most forecasts fail in their attempts to rationalise the past to extrapolate the future.
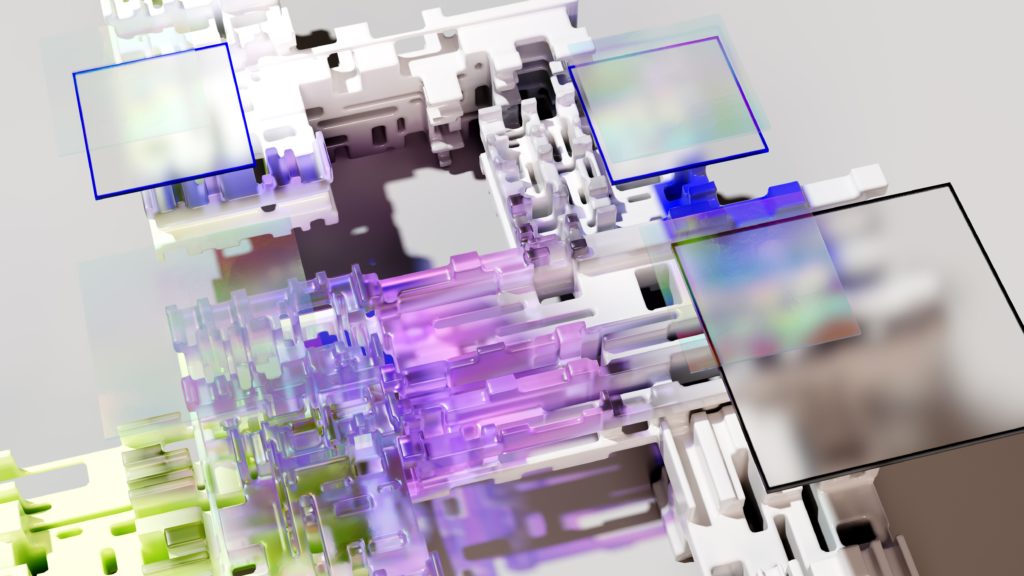
The chaos theory thrives on randomness. At this stage, technology forecasts show a product can create a buzz, boost the status quo or quickly fade into oblivion. Technology develops mathematical models that utilise neural networks to recognise patterns in data sets. The model acts as a foundation for any trends as chaos theory is highly fluid.
Training of the neural network is constant to detect basic technological patterns and validate the forecasts. For any predictions to count from such a framework, quality valid data must be processed and evaluated. However, the algorithms may contain bias due to automation, and this results in misleading trends
Simulations and Scenarios
Scenarios examine the interaction of complex forces that create uncertain futures by using alternative futures to solve an existing problem. For technology forecasting, scenarios explore the path followed by technologies while being rolled out to the masses. It encompasses engineering, applicability, and economic viability. This is unlike back-casting which starts from the future, to the present, and to the future before making any objective assertions. A combination of the two technological forecasting complements each other as scenarios would provide future alternatives while back-casting gains insights for conclusions.
Simulations induce an imaginary perspective of future realities. It’s one of the best technology trends that uses Machine Learning and other methods to gauge anticipated outcomes. Simulations often use live people to find reactionary tactics and plan accordingly.
The future is here
While technology application is vast, ethical guidelines must be followed. Some technological trends such as DNA alternation raise serious moral and existential issues.
Perhaps, to what extent will technology influence the future?